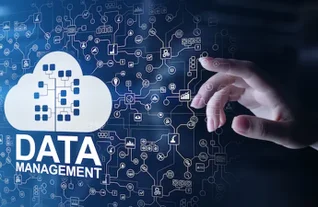
In the digital age, data have become an asset for organizations across various sectors. However, the quality of data is crucial for effective decision-making, accurate insights, and successful business operations. While technological advancements play a significant role in data management, it is essential to recognize the substantial impact humans have on data quality. This article explores the human factors that influence data quality and emphasizes the significance of data governance and individual responsibility in maintaining high-quality data.
Data Entry and Collection:
Data quality issues often originate from errors and inconsistencies during the data entry and collection process. Humans are responsible for inputting data into systems, and even minor mistakes can significantly impact data quality. Illegible handwriting, incorrect interpretations, and data entry errors can introduce inaccuracies that propagate throughout the data lifecycle. Proper training, standardized procedures, and quality control measures are crucial for minimizing these issues.
Lack of Attention to Detail:
Inaccuracies and inconsistencies can arise when individuals fail to pay attention to detail during data manipulation, transformation, and analysis. Human errors such as typographical mistakes, data truncation, and incorrect calculations can lead to misleading results. Organizations must foster a culture that promotes scrutiny of data, encourages double-checking, and emphasizes the importance of accuracy.
Bias and Subjectivity:
Human biases and subjectivity can significantly impact data quality. Biases can be conscious or unconscious, and they can skew data collection, interpretation, and decision-making processes. Biased sampling methods, subjective data interpretation, or deliberate manipulation can compromise the integrity of data. To mitigate these issues, organizations should implement diversity and inclusion initiatives, provide training on bias awareness, and encourage diverse perspectives in data-related tasks.
Insufficient Data Governance:
Data governance refers to the overall management framework and processes that ensure data quality, integrity, and security. Insufficient data governance practices, such as the lack of clear data ownership, inadequate data documentation, and inconsistent data standards, can result in poor data quality. Establishing robust data governance frameworks, implementing data quality checks, and defining data stewardship roles are essential for maintaining data accuracy and consistency.
Data Privacy and Security:
Human actions can compromise data quality when data privacy and security measures are not appropriately implemented. Unauthorized access, data breaches, or negligent handling of sensitive information can lead to data corruption, loss, or unauthorized modifications. Organizations must prioritize data security through measures such as access controls, encryption, regular data backups, and employee training on data protection.
Lack of Data Literacy and Training:
Data literacy, which involves the ability to read, understand, analyze, and communicate data effectively, is crucial for maintaining data quality. Insufficient data literacy among employees can result in misinterpretation, misuse, or misrepresentation of data. Organizations should invest in comprehensive data literacy programs and provide continuous training to ensure employees understand the importance of data quality and possess the necessary skills for data-related tasks.
Furthermore, it is important to highlight that there are certain positive aspects of data quality that would not be possible without human involvement. While humans can introduce errors, biases, and inconsistencies, their active participation is also instrumental in improving and enhancing data quality. Here are a few positive contributions humans bring to the table:
Data Validation and Contextual Understanding:
Humans possess the ability to validate data by applying contextual knowledge and critical thinking. They can identify inconsistencies, anomalies, or outliers that automated processes might overlook. Through their understanding of the broader context, humans can discern when data seem implausible or contradictory, leading to the detection and rectification of potential errors.
Data Cleansing and Enrichment:
Human involvement is essential in the process of data cleansing and enrichment. They can manually review and correct data inaccuracies, ensuring that the information is reliable and consistent. Humans can also enrich the data by adding relevant metadata, context, or additional details that automated systems may not capture. This contributes to a higher level of data quality and comprehensiveness.
Complex Data Interpretation:
While machines excel at processing large volumes of data, humans possess unique cognitive abilities that allow them to interpret complex data. They can understand subtle nuances, interpret unstructured data, and apply critical thinking to derive meaningful insights. Humans play a crucial role in analyzing data in the context of real-world scenarios, identifying patterns, and making informed decisions based on their domain expertise.
Ethical Considerations and Judgment:
Human involvement is necessary for making ethical considerations and exercising judgment in data-related matters. While algorithms and automated systems can assist in decision-making, humans are responsible for assessing the ethical implications, considering the potential biases, and making value-based judgments. This human oversight ensures that data quality is not compromised at the expense of ethical standards and societal considerations.
Continuous Improvement and Adaptability:
Humans are adept at recognizing areas of improvement and adapting to changing data requirements. They can identify emerging data quality issues, propose process enhancements, and adjust data governance frameworks accordingly. Humans possess the flexibility and creativity to refine data quality practices, ensuring that they remain effective in evolving technological landscapes.
The human impact on data quality cannot be overlooked in today's data-driven world. From data entry to analysis, humans play a significant role in maintaining data accuracy, consistency, and integrity. By implementing robust data governance practices, fostering a culture of attention to detail, addressing biases, and promoting data literacy, organizations can mitigate human-induced data quality issues. Recognizing an individual responsibility and providing the necessary tools and training will ultimately result in improved data quality, leading to more reliable insights and better decision-making. Although humans can introduce challenges to data quality, they also bring unique capabilities that positively impact data quality. Their validation skills, ability to cleanse and enrich data, interpretation capabilities, ethical considerations, and adaptability are invaluable for ensuring high-quality data. A balanced approach that combines human expertise with automated systems is crucial for achieving optimal data quality outcomes.
Add comment
Comments